The Forecaster: Using Machine Learning to Predict Energy Consumption and Generation
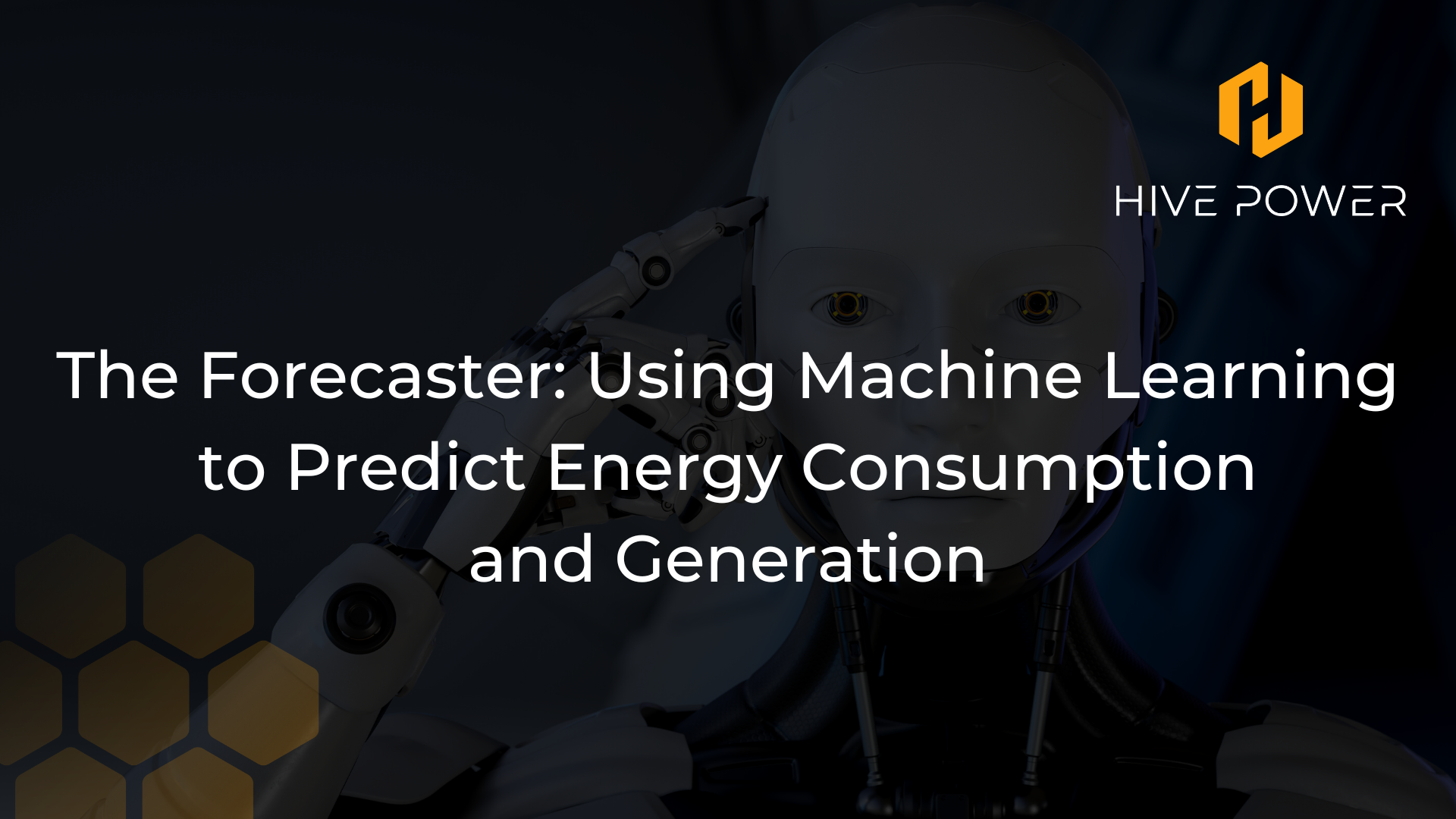
"It's hard to make predictions - especially about the future."- Robert Storm Petersen
Predicting the future might well be hard, but it's often necessary to run the business. Energy forecasting is of primary importance in day-to-day market operations. Short-term forecasting generally involves forecast horizons that range from a few minutes to a few days ahead. Energy Suppliers and Distribution System Operators benefit from accurate predictions of power demand and generation because they can optimally orchestrate their flexible assets to achieve their business goals.
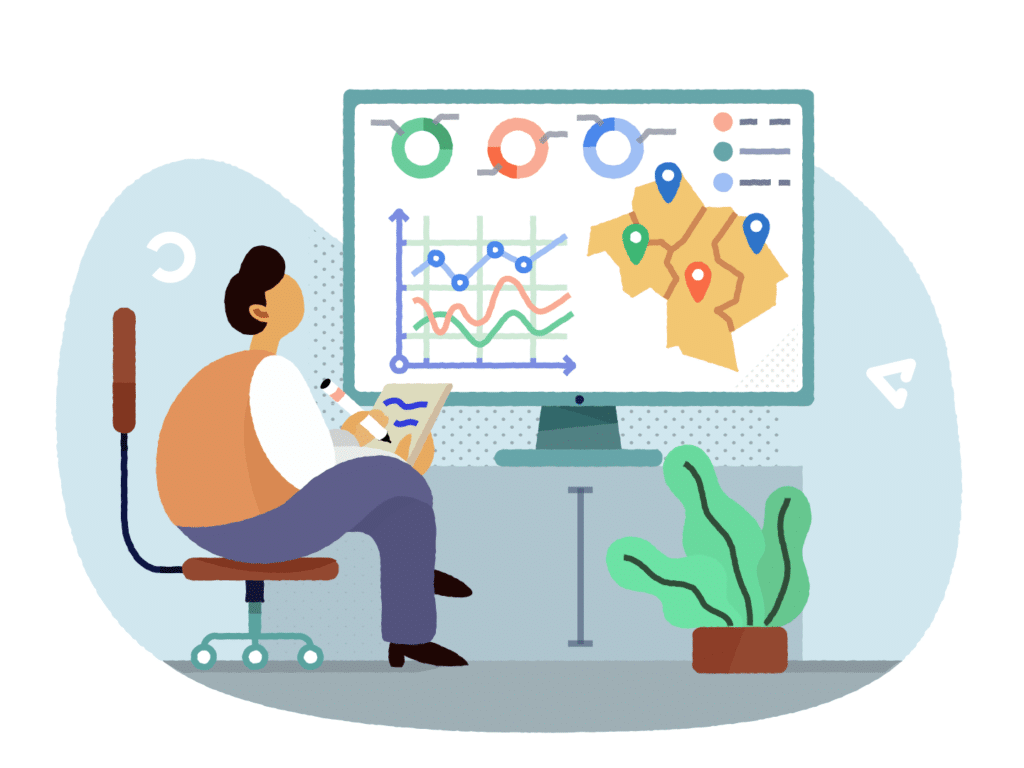
Hive Platform's Forecaster module computes short-term stochastic forecasts of aggregated energy consumption and PV generation, using the most advanced machine learning methods available. Stochastic means that we not only predict the "shape" of the load curve over time but also calculate confidence intervals around this curve. Prediction intervals are essential when it comes to risk management applications, such as predicting the daily or monthly peak of consumption and efficiently activating peak shaving mechanisms.
How does it work?
There is no crystal ball (unfortunately). Instead, the Hive Platform's Forecaster predicts future consumption and generation by learning what happened in the past and using information available from the future. The Forecaster consists of an ensemble of machine learning models trained on and continuously re-informed by a rich dataset.
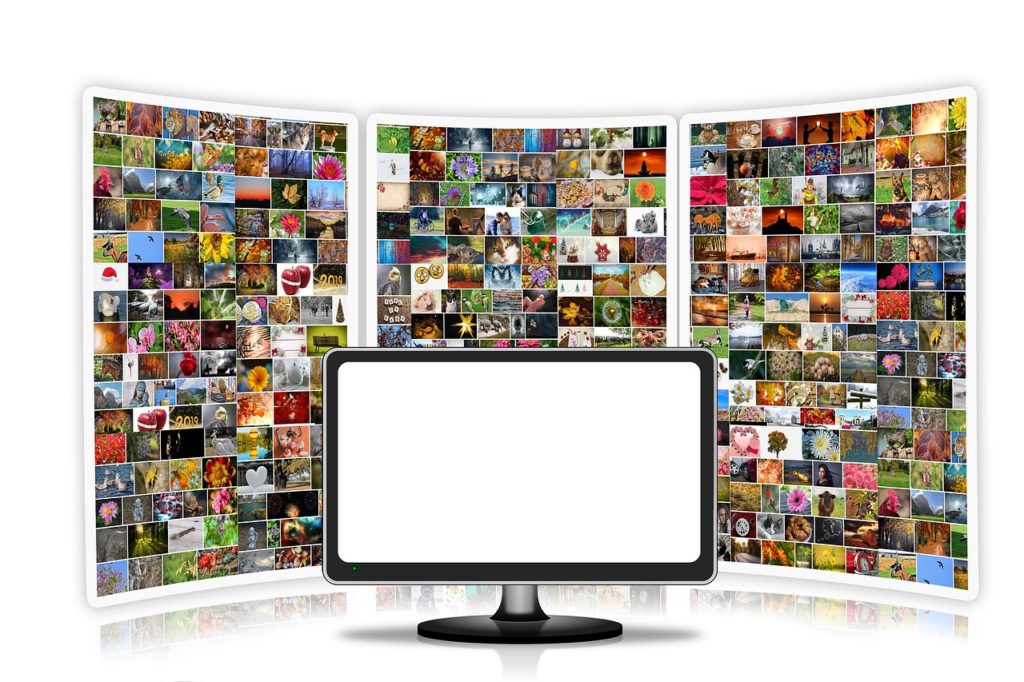
The data fed into these models includes the power signal itself, numerical weather predictions (temperature, solar radiation, wind speed and direction, humidity, and heaps of other parameters), seasonality information (the time of the day, the day of the week, the month of the year, etc.), public holidays, school holidays, and custom events (lockdowns, white nights, major events, religious events, etc.).
Weather is king
Weather is by far the most important external factor affecting energy consumption and generation. For this reason, Hive Power decided to conduct an internal research study to review and select the most performant numerical weather prediction provider. One important criterion we considered was the availability of historical weather forecasts. Many weather providers do not archive and store their predictions. However, historical weather forecasts are of crucial importance to train an energy prediction model. To be robust and reliable, a model should be trained on the same type of data that will be used at inference time. To understand this point, think of the following. If we trained the model on actual weather observations, the model would learn to trust the weather signals to a certain level. When using this model to predict the future, we would need to replace weather observations with weather predictions, which will not be 100% accurate by definition of prediction. Hence the model will give too much weight to the weather predictions and fool itself into error. Conversely, if we train the model on weather predictions, the model will learn to trust less the weather parameters and commit less error at inference time. Still sceptical? Try out yourself. Some of our most wary scientists did so and found exactly what was expected.
Weather forecast benchmark
We looked at about a dozen different providers, filtered out those that did not tick our boxes, and ended up with five finalists. We then asked them for a sample of their data to build a benchmark. We requested a year's worth of hourly predictions of ground-level temperature and solar radiation generated for a single location at around midnight and covering from 24 to 48 hours ahead, which is the typical forecast horizon of our energy prediction models. We compared these predictions with actual local observations and were stunned to discover Meteomatics' superior performance. In the figures below, we show some results. In Figure 1, we plotted the distribution of the discrepancy between observed and predicted temperature. In Figure 2, we overlay the five distributions for a more convenient comparison. A similar situation was found for the solar radiation parameters. It was then clear to us that Meteomatics numerical weather predictions were the most accurate and well-calibrated.
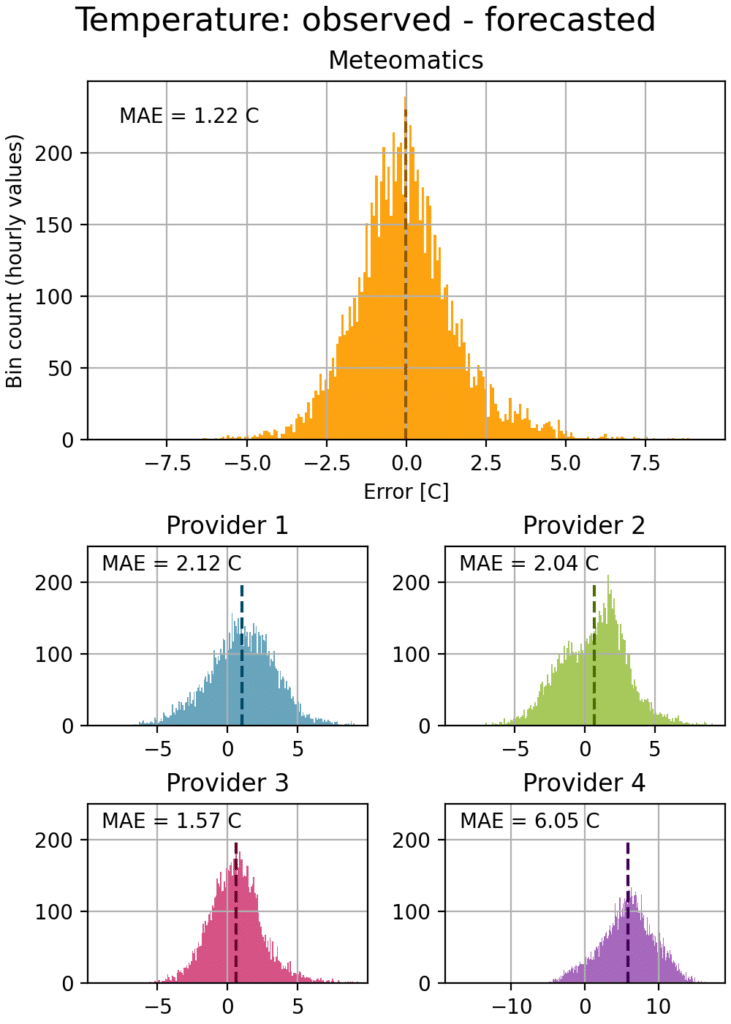
Figure 1 - Distribution of the discrepancy between observed and predicted ground temperature for five different weather providers. The vertical dashed lines indicate the mean of each distribution (only Meteomatics' mean error is centred on zero). The Mean Absolute Error (MAE) is reported on each chart (the lower, the better).
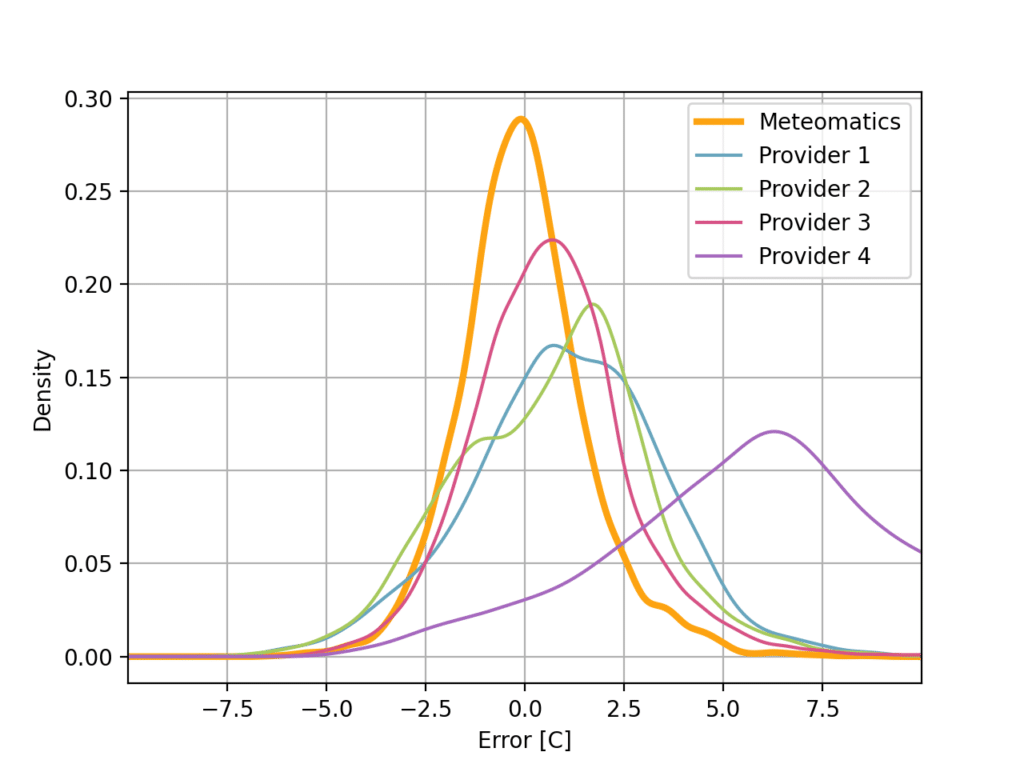
Figure 2 - The same error distributions of Figure 1 overlaid (after estimating their kernel density). Meteomatics' curve is the narrowest and the only one that is zero-centred.
The beginning of a strategic partnership
"We were looking for a one-stop-shop that could provide us with up-to-date and detailed weather data covering Europe, which needed to be conveniently available via a RESTful API. We took quite some time to evaluate several numerical weather prediction providers. It was not an easy choice because of our demanding list of criteria, but Meteomatics checked all the boxes and exceeded our expectations. We were after a provider that covered the whole world, with a focus on Europe and especially the Alps, which are a challenging region when it comes to high-resolution weather forecasting. It was clear from our own forecast benchmarking exercise that Meteomatics was the most accurate weather forecaster, particularly over the short term. We were pleased to discover the rich plethora of standard and advanced weather parameters that Meteomatics' API offers. We are thrilled to have switched to Meteomatics as our weather data provider of choice, and we foresee a long and fruitful partnership with them.", said Gianluca Corbellini, Managing Director at Hive Power.
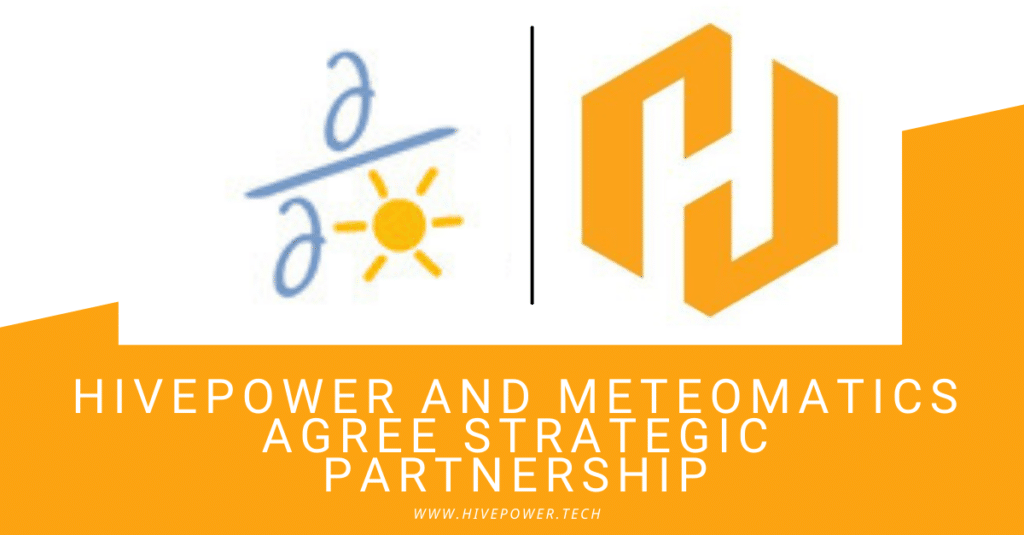
You can read more about this in Meteomatics' latest press release: https://www.meteomatics.com/en/meteomatics-and-hive-power-agree-strategic-partnership/ .Stay tuned for an upcoming webinar (26th of October 2021) where Hive Power and Meteomatics will guide you through an in-depth look at how numerical weather predictions inform energy prediction models.
Comments